LIBRARY
Hierarchical Weight Optimization Design of Aircraft Power Electronics Systems using Metaheuristic Optimization Methods
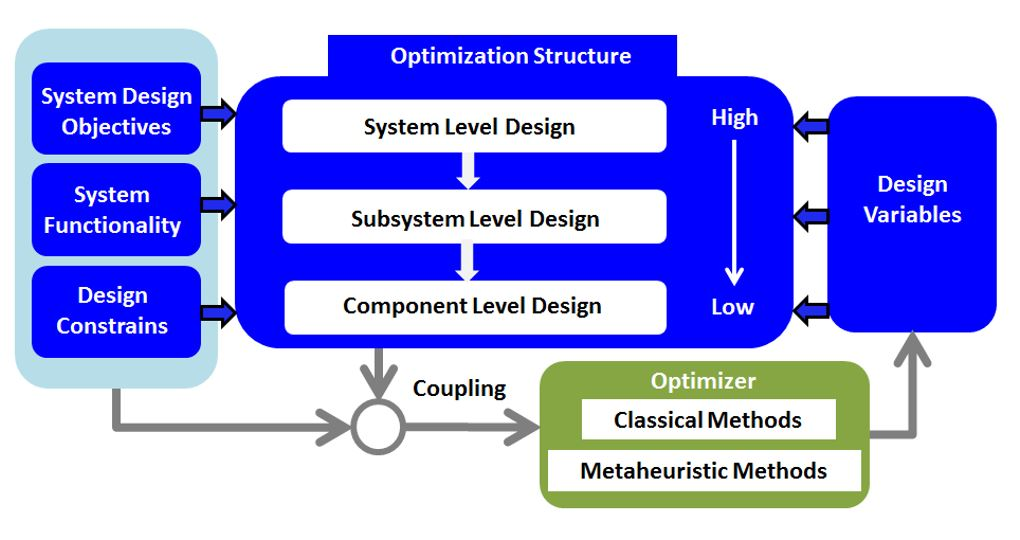
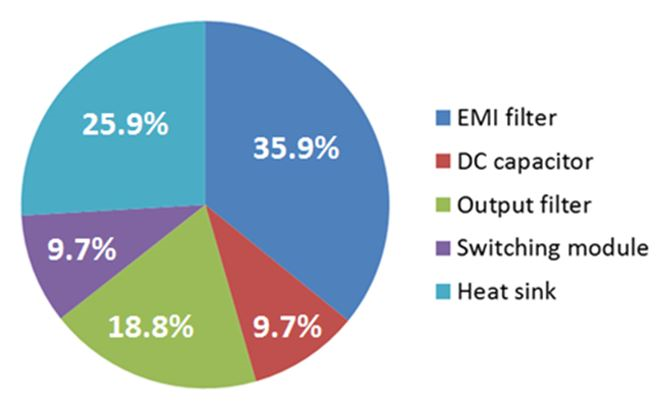

Our library includes the Center's technical papers published in conference and journal papers, presentations, recordings, thesis and dissertations produced by graduates, and a research volume series compiled by CPES faculty.
Visit Resources Visit ResearchOne of the Center's missions is to develop and education program that promotes multi-disciplinary, team-driven, and systems-oriented training to CPES students, as well as provide continuing education opportunities to our industrial partners.
Visit EducationCPES continuously releases news regarding our conferences, faculty, and students. We also provide a list of events for webinars, conferences, and many other facets!
Visit News/EventsCongratulations to the following students who were acknowledged by APEC ...
Qihao Song worked successfully under the close guidance of CPES Associat...
The Applied Power Electronics Conference and Exposition (APEC) focuses o...
CPES Industry Webinars offer engineers from member companies the opportu...
Taking place in Blacksburg, VA on the main campus of Virginia Tech, our ...
CPES Industry Webinars offer engineers from member companies the opportu...
The CPES Industry Consortium cultivates connectivity among researchers in academia and industry, and creates synergy within the network of industry members. Our industry consortium is the backbone of our strong technology transfer success.
Visit IndustryThe Center for Power Electronics Systems (CPES), with annual research expenditures of $6-7 million dollars, is dedicated to improving electrical power processing and distribution that impact systems of all sizes – from battery-operated electronics to vehicles to regional and national electrical distribution systems.
Learn About CPESThe Jobs/Internship Openings page permits anyone from a CPES Member company to post open positions. The person posting can provide supporting information including the position title and description, location, application link, etc. The notices are reviewed and posted where they are accessible to anyone who creates a free account on the CPES website.
Jobs Postings